Pharmacovigilance
Automation
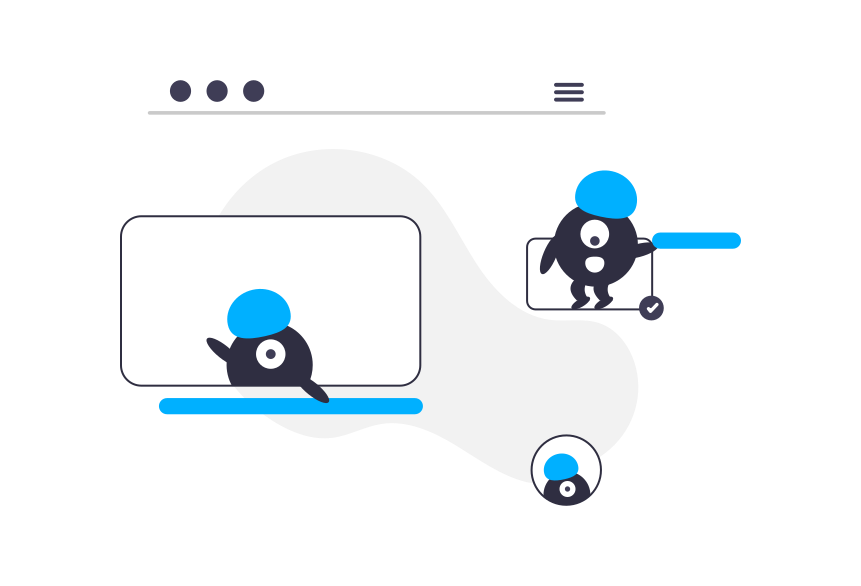
Revolutionizing Pharmacovigilance: Automated Adverse Reaction Detection and Dashboard Creation
Overview:
A leading pharmaceutical company committed to patient safety and drug efficacy sought to enhance its pharmacovigilance efforts. The company recognized the need for a more efficient and accurate adverse reaction detection system. They partnered with Dillify, a trailblazing analytics company, to develop an automated solution leveraging Natural Language Processing (NLP).
Challenges:
1. **Data Volume:** The pharmaceutical company received vast volumes of medical reports, making manual adverse reaction identification labor-intensive and error-prone.
2. **Data Variability:** Adverse reaction descriptions varied widely, posing challenges for rule-based systems.
3. **Timeliness:** Rapid identification of adverse reactions was crucial to expedite regulatory reporting and patient safety.
Solution:
1. **Data Collection and Preprocessing:
The Dillify team collaborated closely with the pharmaceutical company to collect and preprocess medical reports, extracting relevant information while maintaining data privacy.
2. **Natural Language Processing (NLP):
NLP algorithms were applied to analyze unstructured text in medical reports. These algorithms detected adverse reactions, including rare and emerging ones, with high accuracy.
3. **Dashboard Creation:
Dillify designed a user-friendly dashboard that presented adverse reaction insights in real-time. The dashboard included visualization tools for easy interpretation.
4. **Machine Learning Models:
Machine learning models were incorporated to continuously improve detection accuracy by learning from newly reported adverse reactions.


Results:
The implementation of automated adverse reaction detection and the creation of a dashboard yielded remarkable results:
- **Enhanced Efficiency:** Manual adverse reaction identification time reduced significantly, enabling quicker regulatory reporting.
- **Accuracy:** The NLP-based system detected adverse reactions with higher accuracy, reducing false positives.
- **Real-Time Insights:** The dashboard provided real-time adverse reaction data, empowering faster decision-making.
- **Adaptability:** Machine learning models adapted to emerging adverse reactions, ensuring ongoing accuracy.
Monitoring and Ongoing Improvement:
The pharmaceutical company established a robust monitoring framework to ensure continuous improvement:
- **Regular Model Updates:** NLP models were updated regularly to adapt to evolving medical terminology.
- **Feedback Loop:** Continuous feedback from pharmacovigilance experts improved system performance.
- **Data Security:** Stringent data security measures protected sensitive patient information throughout the process.
Conclusion:
The collaboration between the pharmaceutical company and Dillify resulted in a groundbreaking pharmacovigilance automation model. By leveraging NLP, the company achieved rapid and accurate adverse reaction detection while the dashboard provided real-time insights for timely actions. This case study exemplifies the transformative power of data-driven analytics in enhancing patient safety and regulatory compliance within the pharmaceutical industry.
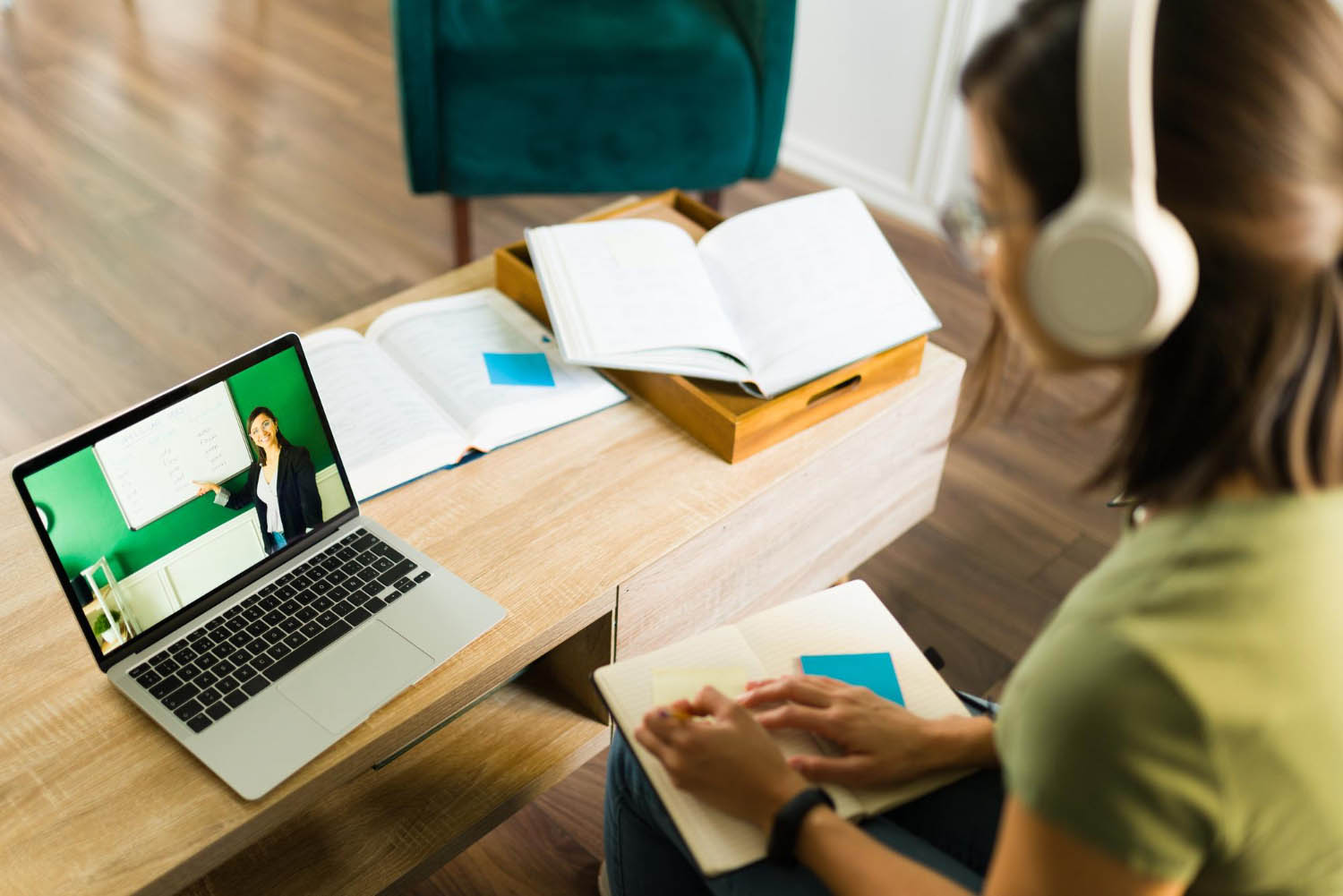