Automated Xray
Diagnostics
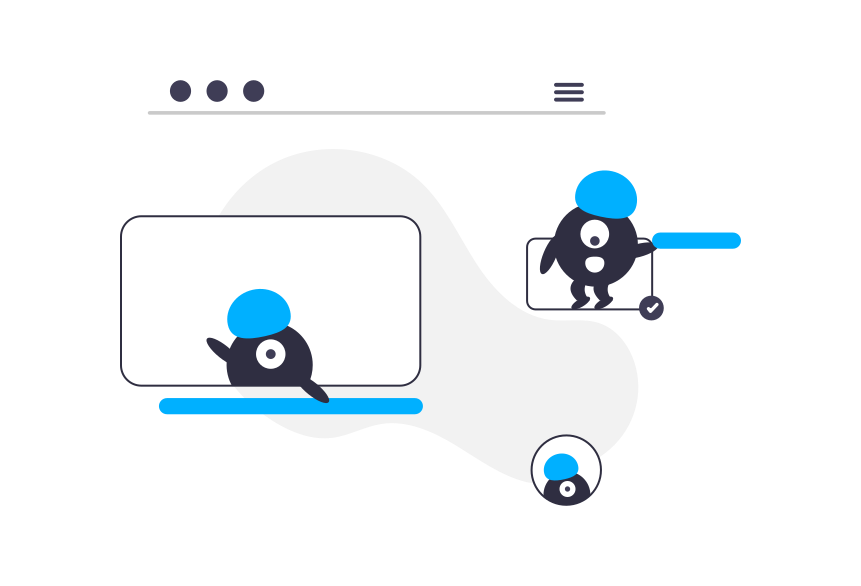
Transforming Healthcare: Automated X-Ray Diagnosis and Reporting with Computer Vision
Overview:
A leading healthcare institution committed to enhancing patient care and efficiency sought to revolutionize its radiology department. Facing increasing workloads and the need for rapid diagnostics, the institution partnered with Dillify, a pioneer in data-driven healthcare solutions. Together, they developed an innovative system for automated X-ray diagnosis and reporting using cutting-edge Computer Vision (CV) models.
Challenges:
1. **Workload Overload:** Radiologists were inundated with X-ray images, leading to potential delays in diagnoses and reporting.
2. **Human Error:** Manual interpretation of X-rays was susceptible to human errors, potentially impacting patient outcomes.
3. **Timely Reporting:** Ensuring rapid diagnosis and reporting was crucial for timely patient care and treatment decisions.
Solution:
1. **Data Collection and Augmentation:
Dillify collaborated closely with the healthcare institution to collect a diverse dataset of X-ray images. The data was augmented to improve model robustness.
2. **Computer Vision Models:
Advanced CV models were developed to detect abnormalities and anomalies in X-ray images. These models included Convolutional Neural Networks (CNNs) and deep learning architectures.
3. **Automated Reporting:
A reporting system was integrated with the CV models, generating detailed diagnostic reports for each X-ray image.
4. **Real-Time Results:
The system provided real-time diagnostic results, reducing turnaround time for patient reports.


Results:
The implementation of automated X-ray diagnosis and reporting using CV models delivered remarkable results:
- **Efficiency:** The system significantly reduced the workload of radiologists, allowing them to focus on complex cases.
- **Accuracy:** CV models achieved high accuracy in detecting abnormalities, minimizing the risk of misdiagnosis.
- **Rapid Reporting:** The real-time reporting system ensured that patients received timely diagnostic reports for prompt treatment.
- **Quality of Care:** Improved accuracy and speed enhanced the overall quality of patient care.
Monitoring and Ongoing Improvement:
The healthcare institution established a rigorous monitoring framework to maintain the system's effectiveness:
- **Regular Model Updates:** CV models were updated regularly to adapt to evolving medical imaging standards.
- **Feedback Integration:** Radiologists' feedback played a crucial role in fine-tuning the models and reporting system.
- **Data Security:** Stringent data security measures ensured patient confidentiality and compliance with healthcare regulations.
Conclusion:
The partnership between the healthcare institution and Dillify resulted in a game-changing solution for automated X-ray diagnosis and reporting. Leveraging state-of-the-art CV models, the institution achieved faster, more accurate diagnostics, ultimately improving patient care and outcomes. This case study exemplifies the transformative potential of data-driven analytics in healthcare, particularly in radiology departments.
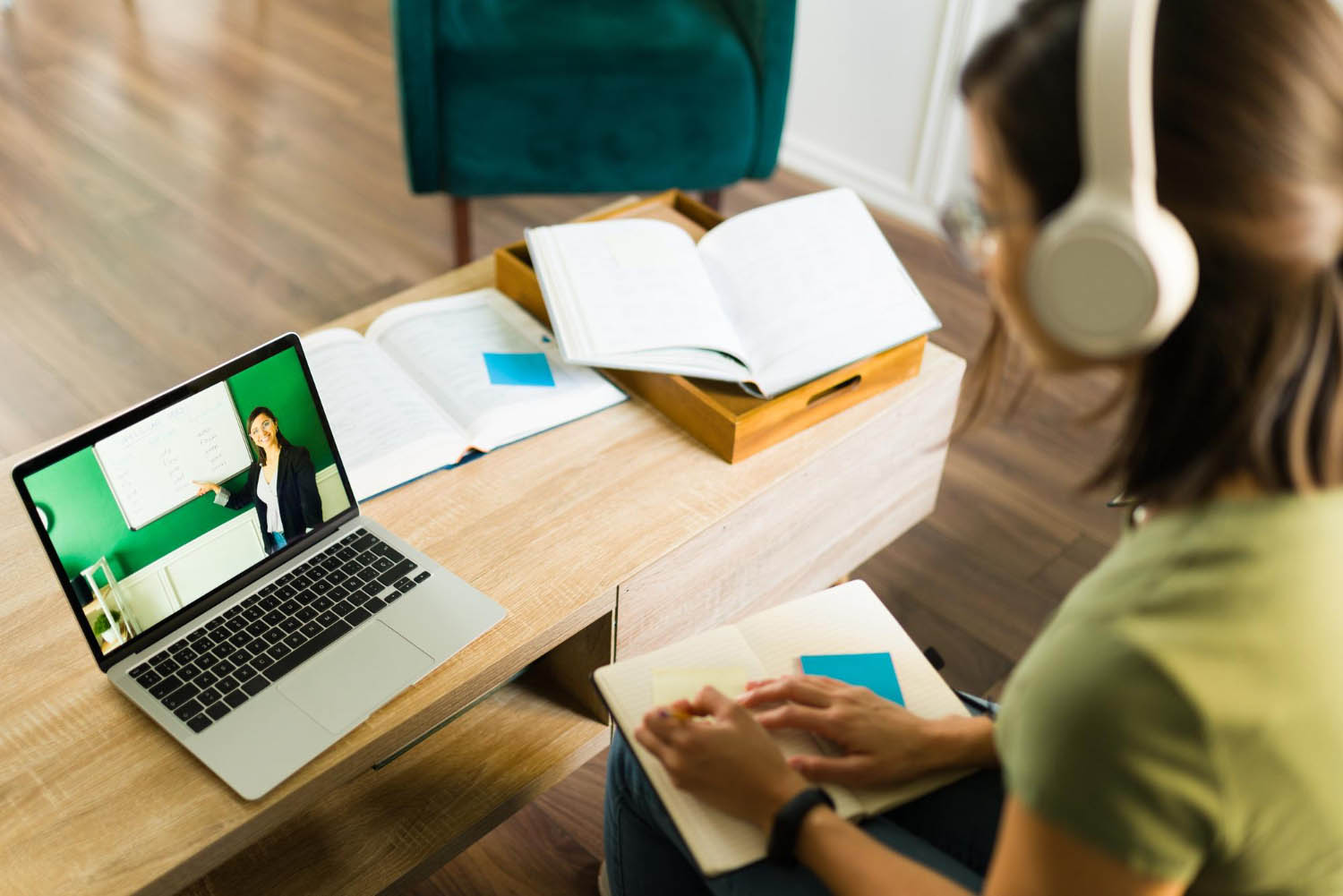