Ride Demand &
Supply Prediction
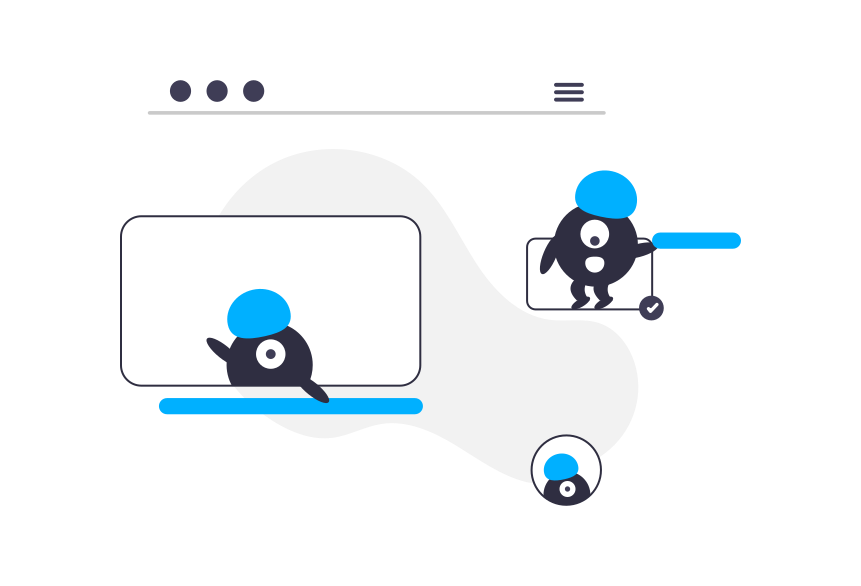
Enhancing Mobility: A Ride/Cab Demand and Supply Prediction Model for a Leading Middle Eastern Transportation Provider
Overview:
A prominent Middle Eastern transportation provider sought to optimize its ride/cab services by predicting demand and efficiently managing its supply chain. They partnered with Dillify, a leader in data-driven solutions, to develop an advanced prediction model. This model aimed to forecast ride demand, allocate resources effectively, reduce waiting times for passengers, and improve overall customer satisfaction.
Challenges:
1. **Fluctuating Demand:** The transportation provider faced fluctuating ride demand due to factors like weather, events, and time of day, making it challenging to allocate resources optimally.
2. **Resource Allocation:** Ensuring that an adequate number of cabs were available in high-demand areas while avoiding oversupply in low-demand regions was a complex task.
3. **Customer Experience:** Passengers often experienced longer waiting times during peak hours, leading to dissatisfaction.
Solution:
1. **Data Collection and Processing:
Dillify collaborated closely with the transportation provider to collect historical ride data, weather data, event schedules, and traffic data. This data was processed and integrated into the prediction model.
2. **Machine Learning Model Development:
The Dillify data science team developed a machine learning model based on advanced time-series forecasting techniques. The model could predict ride demand for various time intervals and locations.
3. **Supply Chain Optimization:
Intelligent algorithms were implemented to optimize resource allocation. The model determined the ideal number of cabs to allocate to different areas in real-time.


Results:
The implementation of the Ride/Cab Demand and Supply Prediction Model delivered impressive results:
- **Reduced Waiting Times:** Passengers experienced significantly shorter waiting times during peak demand hours.
- **Optimized Resource Allocation:** The transportation provider achieved cost savings by allocating cabs more efficiently.
- **Customer Satisfaction:** Improved service quality and reduced waiting times led to higher customer satisfaction and loyalty.
- **Data-Driven Decision-Making:** The model empowered the transportation provider with data-driven insights for strategic planning and expansion.
Future Expansion:
The transportation provider and Dillify are now exploring opportunities for further enhancement:
- **Integration of Real-Time Data:** Leveraging real-time traffic and event data for more accurate predictions.
- **Multi-Modal Transportation:** Extending the model to optimize the entire transportation network, including taxis, buses, and ride-sharing services.
- **Sustainability Initiatives:** Exploring ways to reduce environmental impact by optimizing routes and vehicle allocation.
Conclusion:
The collaboration between the Middle Eastern transportation provider and Dillify resulted in a transformative Ride/Cab Demand and Supply Prediction Model. By harnessing the power of data and machine learning, the transportation provider enhanced mobility, reduced passenger wait times, and improved overall customer satisfaction, setting a new standard for transportation services in the Middle East.
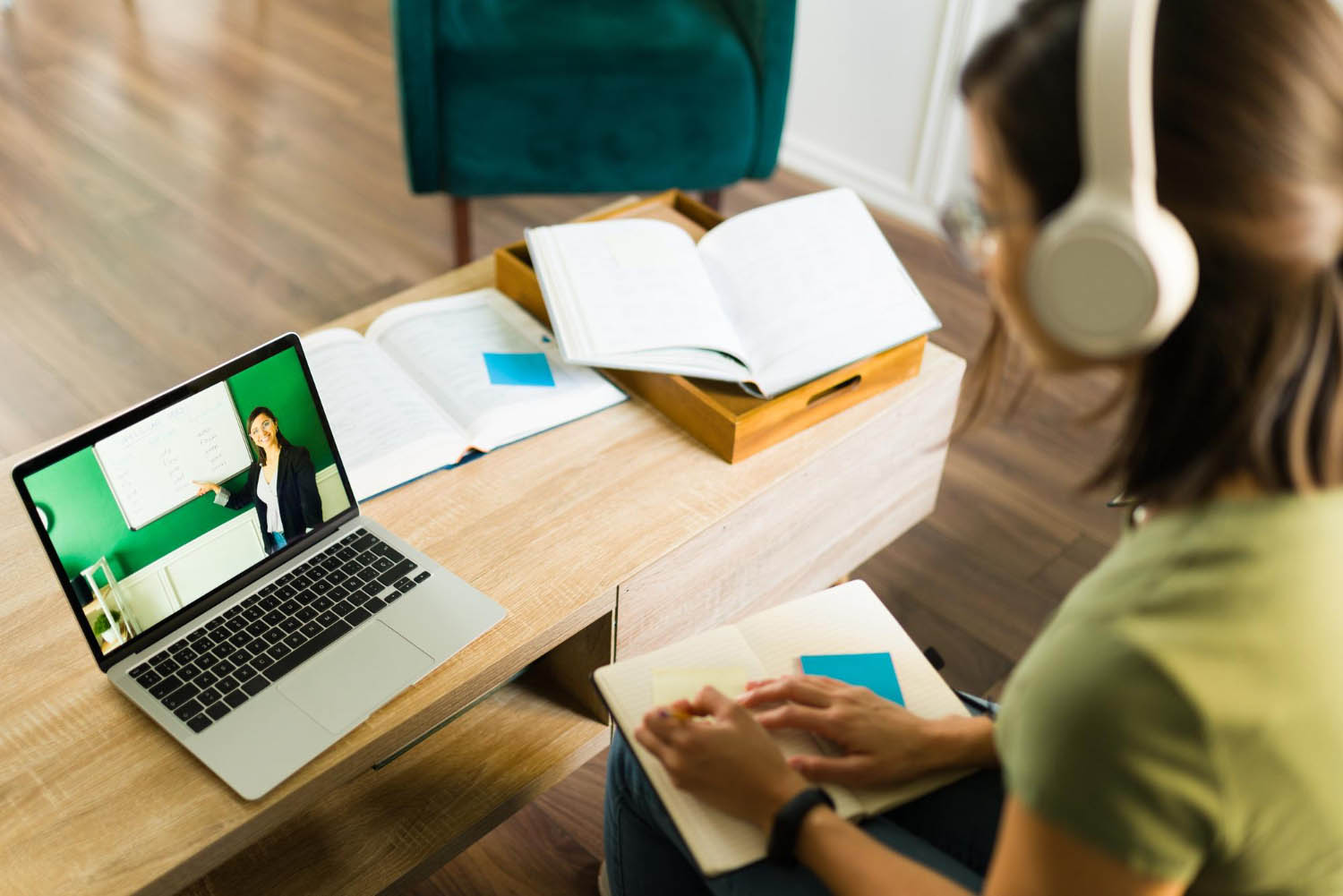