Personalized Recommender
Engine for upsell
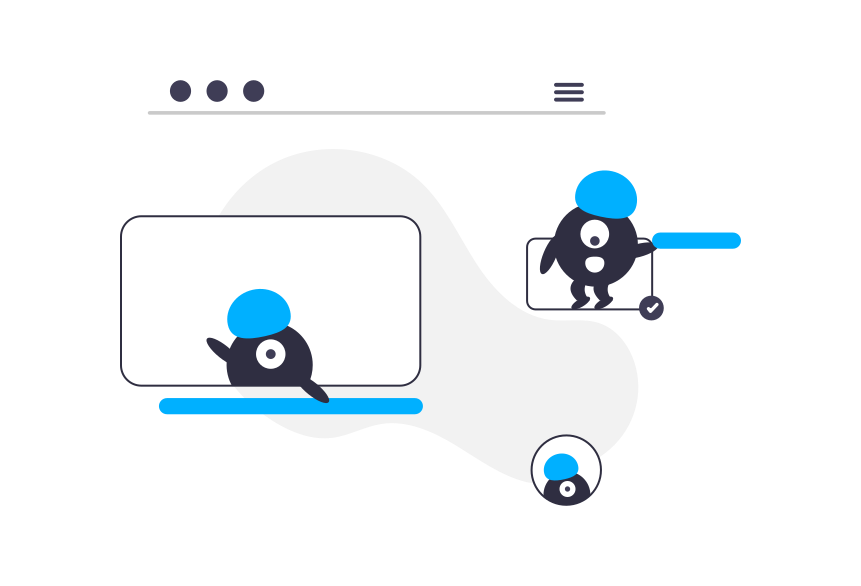
Enhancing User Experience: Building a Personalized Recommendation Engine for an IT Giant
Overview:
An industry-leading IT giant, known for its diverse range of products and services, recognized the need to deliver a personalized user experience. To achieve this, the company partnered with Dillify, a leader in data-driven solutions, to develop a state-of-the-art recommendation engine. The goal was to offer tailored content and product suggestions to users, thereby increasing engagement and customer satisfaction.
Challenges:
1. **Vast Content Catalog:** The IT giant had an extensive catalog of products, services, and content, making it challenging for users to discover relevant items.
2. **User Engagement:** Improving user engagement and retention was a top priority. The company wanted to keep users coming back for more.
3. **Personalization:** Generic recommendations were no longer effective. The company sought to provide personalized suggestions based on user behavior and preferences.
Solution:
1. **Data Collection and Integration:
Dillify worked closely with the IT giant to gather and integrate user data, including browsing history, search queries, and previous interactions with the platform.
2. **Machine Learning Algorithms:
Advanced machine learning algorithms, including collaborative filtering, content-based filtering, and hybrid models, were implemented to power the recommendation engine.
3. **Real-Time Personalization:
The recommendation engine provided real-time personalization, offering content and product suggestions as users navigated the platform.


Results:
The implementation of the personalized recommendation engine delivered impressive results:
- **User Engagement:** User engagement increased significantly as users received tailored content and product suggestions.
- **Retention:** The platform experienced higher user retention rates, with users returning more frequently.
- **Revenue Growth:** Personalized recommendations led to increased product sales and content consumption, contributing to revenue growth.
- **Enhanced User Experience:** Users praised the platform's improved usability and the relevance of suggested items.
Monitoring and Continuous Improvement:
The IT giant established a robust monitoring and improvement framework to ensure ongoing success:
- **Feedback Loop:** User feedback played a vital role in refining the recommendation engine and addressing user concerns.
- **Algorithm Updates:** Regular updates to the recommendation algorithms ensured their adaptability to changing user behaviors.
- **Data Privacy:** Stringent data privacy measures protected user information and maintained compliance with regulations.
Conclusion:
The collaboration between the IT giant and Dillify resulted in a groundbreaking personalized recommendation engine. By harnessing the power of machine learning and real-time personalization, the company successfully improved user engagement, retention, and revenue. This case study exemplifies the transformative potential of data-driven analytics in delivering exceptional user experiences for technology giants.
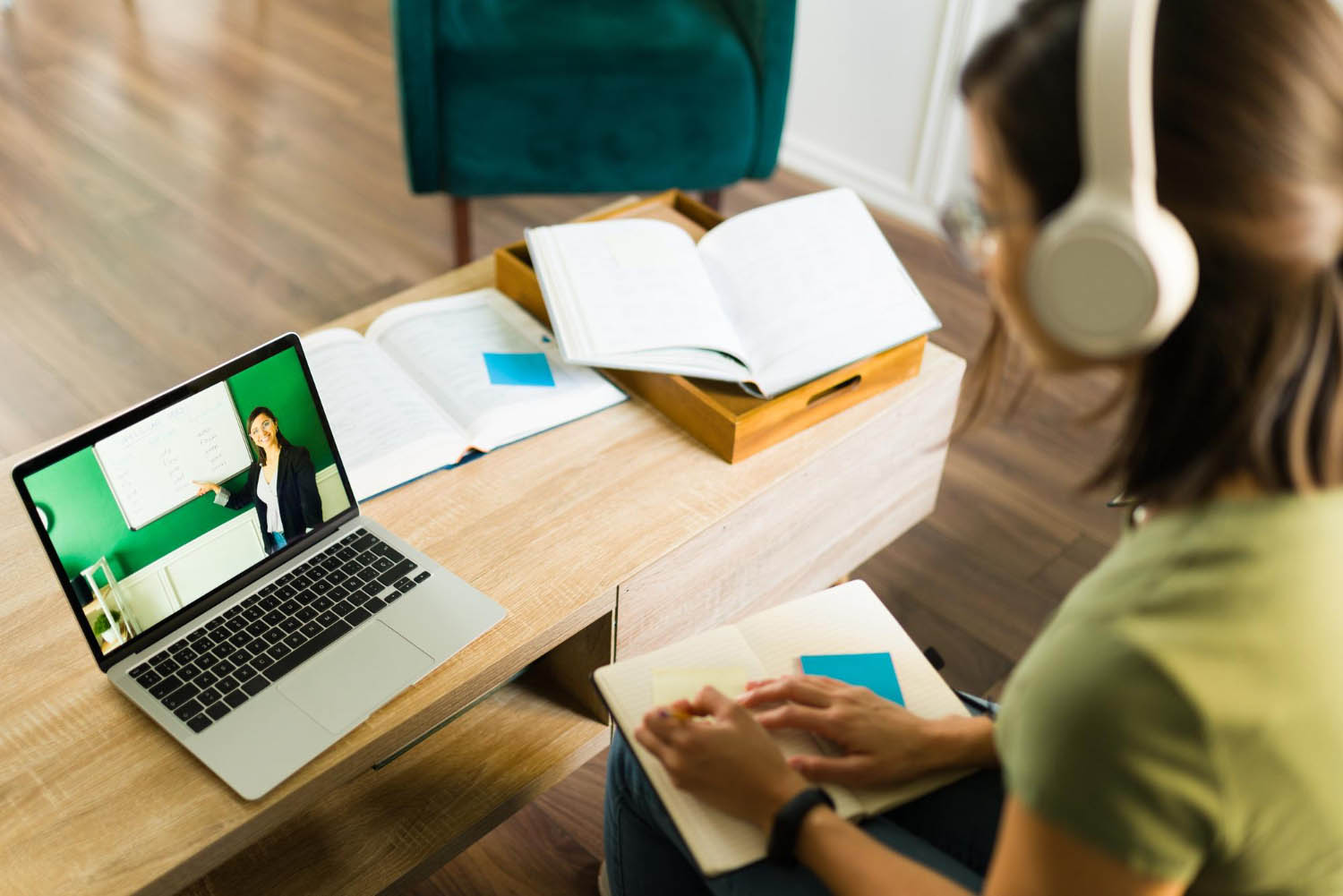