Payment Wallet
Customer Churn
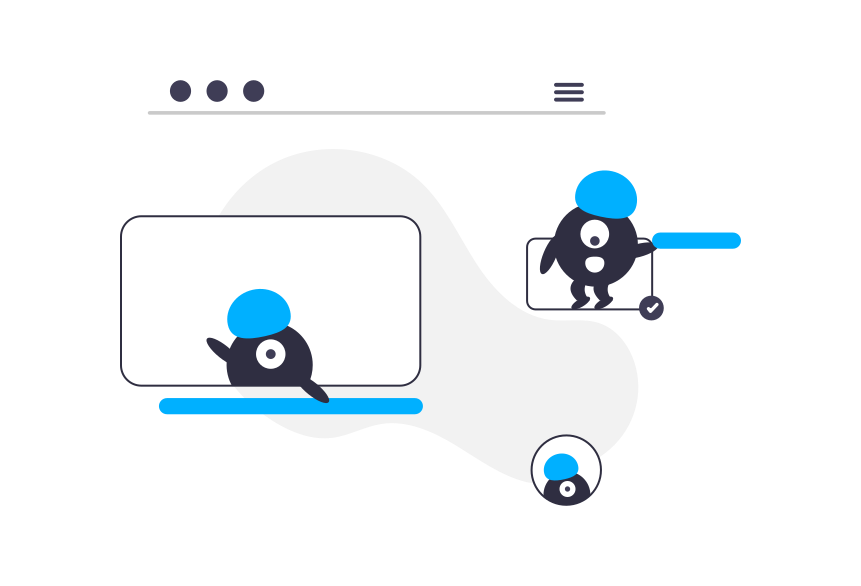
Enhancing Customer Retention: Customer Churn Prediction for India's Largest Payment Wallet
Overview:
India's largest payment wallet, a pioneer in digital payments, aimed to reduce customer churn and enhance user engagement. Faced with increasing competition, the wallet provider collaborated with Dillify, a leading analytics company, to develop a cutting-edge customer churn prediction model.
Challenges:
1. **Rising Competition:** The payment wallet faced fierce competition from other digital payment platforms, increasing the risk of customers switching providers.
2. **Complex User Behavior:** Users exhibited diverse transaction patterns, making it challenging to identify churn indicators accurately.
3. **Retention Strategy:** The wallet provider needed actionable insights to implement effective retention strategies and reduce churn.
Solution:
1. **Data Collection and Preparation:**
Dillify collaborated closely with the wallet provider to collect and preprocess extensive user data, transaction history, and customer interaction records.
2. **Algorithm Selection:**
Dillify recommended a machine learning ensemble approach, combining Random Forest and Recurrent Neural Networks (RNNs) to capture both structured and sequential data patterns.
3. **Model Development:**
Dillify's data science team implemented the chosen algorithms using Python and specialized libraries like TensorFlow and scikit-learn. The model was trained to predict user churn.
4. **Feature Engineering:**
Advanced feature engineering techniques were applied to create new variables and capture complex user behaviors that could predict churn.
5. **Real-Time Integration:**
Dillify collaborated with the wallet provider's IT team to integrate the churn prediction model into the payment platform for real-time assessment and personalized user interventions.
6. **Model Performance Evaluation:**
The model underwent rigorous evaluation using historical data, considering metrics such as AUC-ROC, accuracy, precision, and recall to ensure its effectiveness.
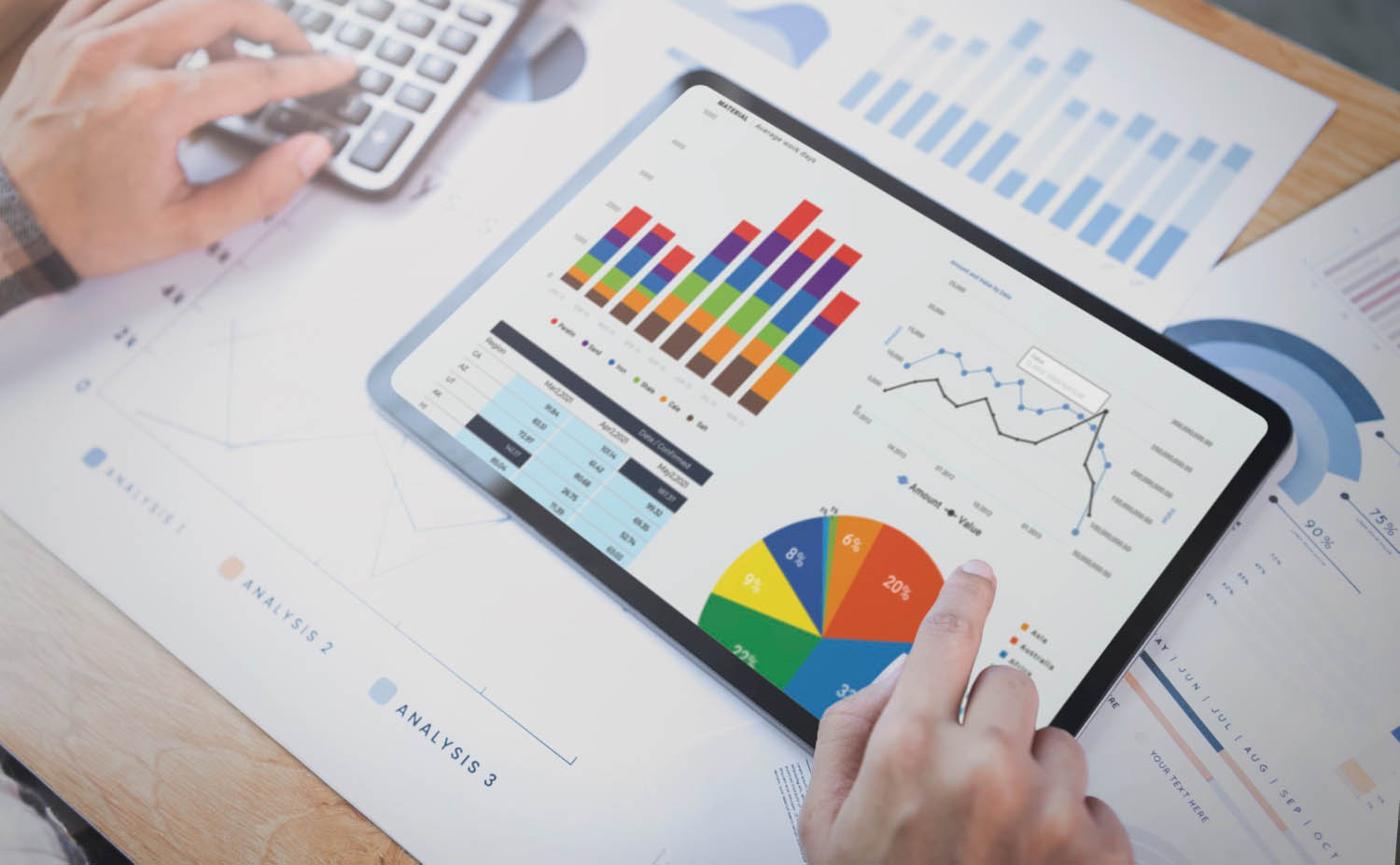
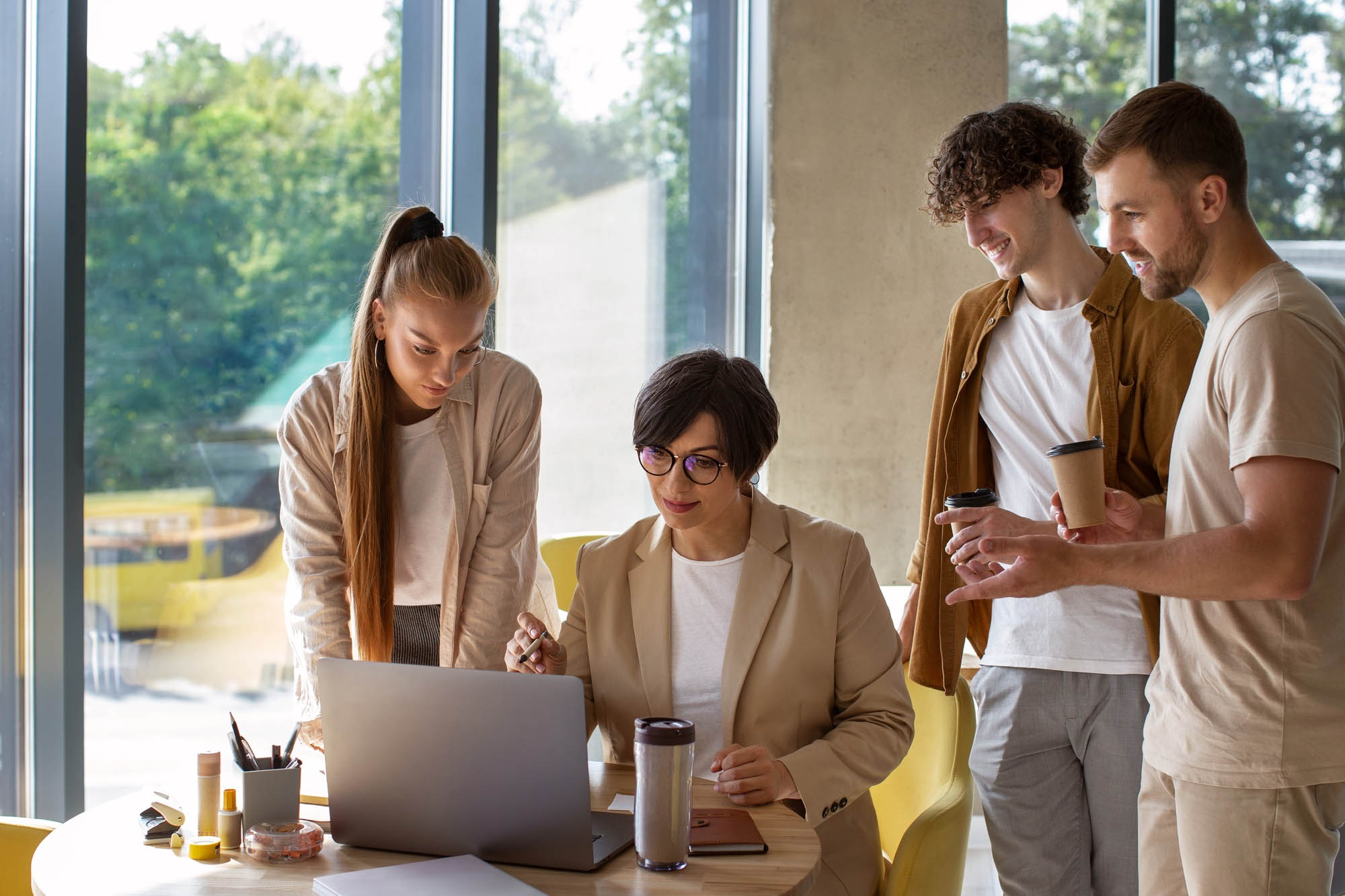
Results:
The implementation of Dillify's customer churn prediction model led to significant outcomes:
- **Churn Reduction:** The model identified potential churners with an accuracy of over 85%, enabling proactive retention efforts.
- **Personalized Engagement:** Real-time integration allowed the wallet provider to engage with users at risk of churn through personalized offers and incentives.
- **User Satisfaction:** By addressing potential issues before users considered switching, the wallet provider improved customer satisfaction.
- **Revenue Growth:** Reduced churn and increased user engagement translated into higher revenue for the payment wallet.
Monitoring and MLOps:
Dillify established a robust monitoring and MLOps framework for continuous model performance:
- **Data Updates:** The model regularly incorporated new user data and transaction records to adapt to evolving user behaviors.
- **Scheduled Retraining:** Frequent model retraining ensured its effectiveness against emerging churn patterns.
- **Feedback Loop:** User feedback and engagement data contributed to model improvement and personalized strategies.
- **Security Measures:** Stringent data security measures preserved user privacy throughout the process.
Conclusion:
The collaboration between the payment wallet provider and Dillify resulted in a highly effective customer churn prediction model. By leveraging advanced analytics, real-time integration, and personalized engagement, the payment wallet reduced churn, enhanced user satisfaction, and drove revenue growth. This case study illustrates the pivotal role of data-driven insights in retaining and delighting customers in the fiercely competitive digital payments landscape.
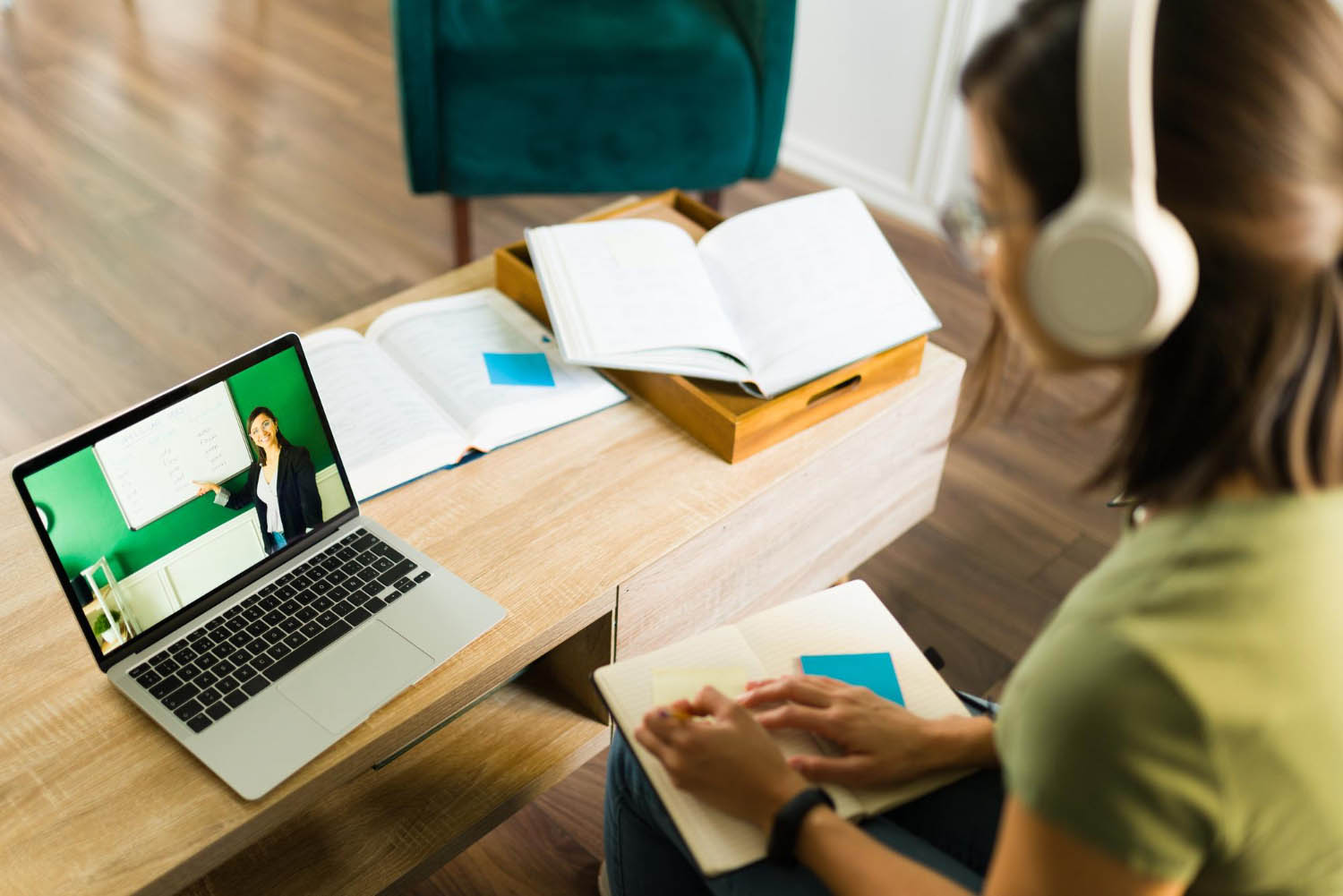